How We Calculated the Return on Investment of Associate Degrees and Certificates
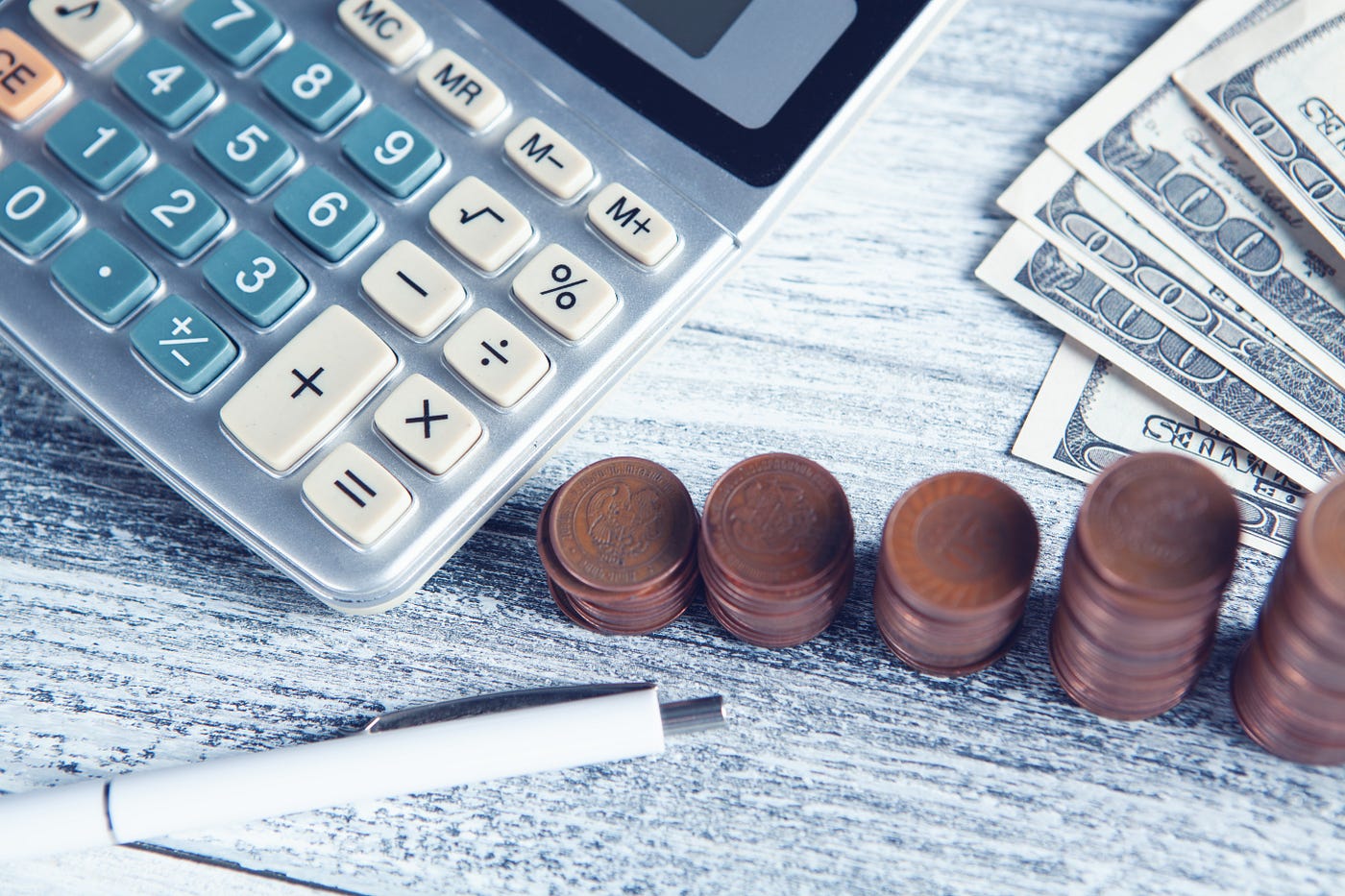
Photo by Sasun Bughdaryan on Unsplash
Our paper Is Community College Worth It? A Comprehensive Return on Investment Analysis calculates the net financial gains (increase in earnings minus education costs) that students can expect from nearly 17,000 associate degrees and certificates across America. This page describes the methodology underlying the report’s estimates of return on investment (ROI) for these sub-baccalaureate credentials.
Returning readers will recognize much of the methodology from FREOPP’s previous work calculating the financial value of bachelor’s degrees and graduate degrees. But because there are differences in the nature of these credentials, as well as the associated data sources, there are some differences in methodology.
The ROI of an associate degree or certificate consists of three components:
- Estimated earnings: The amount that a student who graduates with a certain associate degree or certificate can expect to earn over the course of her life.
- Counterfactual earnings: The amount that the same student would have earned over the course of her life had she never pursued higher education. This figure includes foregone income while she is earning her credential.
- Costs of college: The amount that the student spends on tuition, required fees, and other education-related expenses such as books and equipment. It does not include living expenses, as students must bear these costs regardless of whether they pursue higher education.
ROI is equivalent to estimated earnings minus the sum of counterfactual earnings and college costs. It is the most comprehensive measure of the net financial benefit (or loss) that a student will receive for having earned a particular degree.
As the median age of graduation for associate degrees is 22, the following calculations assume that the student spends two years earning her associate degree and enters the labor force at age 23. Students in certificate programs spend just one year in school and enter the labor force one year earlier, at age 22. The working careers of both types of graduates last until age 64. All cash flows are discounted at a real three percent rate to the year in which the student turns 20. Unless otherwise noted, we adjust all figures to 2020 dollars.
Estimated earnings
The earnings calculation combines two sources of data: the U.S. Department of Education’s College Scorecard by Field of Study and the U.S. Census Bureau’s American Community Survey (ACS). The Scorecard provides information on the median earnings of graduates of over 6,000 undergraduate certificate programs and over 10,000 associate degree programs.
Scorecard earnings are defined as the sum of wage and salary (Form W-2) income and positive self-employment income for individuals who received federal Title IV financial aid. Those who were not employed are excluded from earnings cohorts. Institution-program observations with small cohort sizes are suppressed for privacy reasons, so earnings data are not available for all programs. But the vast majority of sub-baccalaureate students completed a program with reportable data: among students receiving federal financial aid, 87 percent of associate degree completers and 89 percent of undergraduate certificate completers were enrolled in a field of study with reportable earnings data.
The Scorecard’s central limitation is that it only measures student earnings in the first two years after graduation. But since wage profiles tend to be steep during the early career, Scorecard earnings may significantly underestimate the lifetime returns to sub-baccalaureate credentials. For that reason, we augment the Scorecard figures with data from the American Community Survey, provided by the IPUMS USA database at the University of Minnesota.
Conducted by the U.S. Census Bureau, the ACS surveys a random one percent sample of the U.S. population every year, collecting basic demographic data along with information on individuals’ earnings. While the ACS collects data on education levels, it only collects data on field of study for people with at least a bachelor’s degree. ACS reports the education level for people with associate degrees but not the field of study in which their credential was granted. ACS does not report whether respondents hold an undergraduate certificate, but there is an education category for people with one or more years of college credit but no degree; we take this group as a proxy for people with certificates. These limitations will make ROI estimates for associate degrees and certificates somewhat less precise than the estimates for bachelor’s degrees, though outcomes for the average program should be unbiased.
To estimate life-cycle earnings for Scorecard programs, we use a sub-sample of the ACS datasets for the years 2009 through 2019. (We exclude the 2020 sample, as disruptions to data collection during the COVID-19 pandemic may yield unreliable estimates.) The combined sub-sample includes individuals with a bachelor’s degree or higher. We exclude anyone younger than 23 or older than 65. (Earnings are reported for the previous year, so this effectively gives us a sample of incomes for people between ages 22 and 64.) To align with the cohort definition in the Scorecard, we also exclude those currently enrolled in school or college, people who are unemployed or not in the labor force, and anyone with real earnings below $10,000 per year (as this may signal non-employment in the previous year, when earnings were measured).
These exclusions yield a sample of 1.1 million people with associate degrees and 1.7 million people with one or more years of college credit but no degree.
Next, for each age and education level group, we calculate cut points for 250 quantiles. For instance, the 125th quantile (or 50th percentile) for associate degree holders at age 23 is $28,954. But by age 45, 125th quantile earnings for associate degree holders rise to $51,535. Similarly, for people with one or more years of college credit but no degree, 125th quantile earnings start at $24,442 at age 22, but rise to $48,084 by age 45.
After calculating the quantile cut points, we assess where individual Scorecard observations fall on the distribution of earnings for the relevant credential level at the beginning of graduates’ careers. For associate degrees, we compare average Scorecard earnings in the first two years after graduation to ACS earnings for associate degree holders at ages 23 and 24. For certificates, we compare Scorecard earnings to ACS earnings for people with one or more years of college credit but no degree at ages 22 and 23. We identify the closest quantile cut point in ACS for each observation in the Scorecard.
Consider the associate degree in design and applied arts at Northern Virginia Community College (NVCC). Average earnings in the first two years after graduation are $32,970. This corresponds most closely to the 138th quantile of earnings for associate degree holders aged 23 and 24 ($33,089). The 138th quantile approximately equates to the 55th percentile, meaning that earnings for design and applied arts students at NVCC are slightly above the median for all associate degrees.
Weighted by enrollment, the median associate degree program in the Scorecard corresponds to the 127th ACS quantile (roughly equal to the 51st percentile), suggesting that ACS earnings for associate degree holders aged 23 and 24 closely track earnings measured in the Scorecard. The median certificate program corresponds to the 112th ACS quantile (roughly equal to the 45th percentile), suggesting that Scorecard earnings for certificate programs slightly lag ACS earnings for people with one or more years of college credit but no degree, aged 22 and 23. This is expected because the applicable ACS education category includes more than just people with undergraduate certificates; if people who have a year or more of college credit for other reasons (perhaps apprenticeships or industry-recognized certifications) out-earn people who hold postsecondary certificates, then Scorecard earnings would lag the ACS distribution.
We then generate earnings estimates for each Scorecard program at all points during students’ careers by assigning each program the same quantile earnings for each age. For instance, NVCC’s design program corresponds to 138th quantile earnings for associate degree holders at the beginning of graduates’ careers. We assume that graduates’ earnings remain at the 138th quantile for the relevant age group for the rest of their working lives. For associate degree holders aged 35, 138th quantile earnings are $49,140. Therefore, we assign graduates of NVCC’s design program earnings of $49,140 at age 35. We repeat this process for every age group until retirement.
The key assumption underpinning this methodology is that the median graduate of each Scorecard program remains at the same relative place on the earnings distribution throughout her entire career.
Note that this methodology considers returns on the associate degree or certificate only. If a student uses her sub-baccalaureate credential as a stepping-stone for a bachelor’s degree, the returns to that higher degree are not reflected in the estimates here.
Readers of our previous work on ROI may wonder why we use quantiles rather than Z-scores to extrapolate Scorecard earnings using ACS. The quantile method is superior as it more precisely reflects the distribution of earnings at each age. However, using the quantile method effectively requires a very large sample size. The earnings extrapolation process for bachelor’s and graduate degrees relies on much smaller samples of ACS respondents broken out by field of study, so the Z-score method is more appropriate. But the sample size for each age-education group in ACS is much larger — always greater than 10,000 — so it is possible to use the quantile method.
The calculations yield estimates of typical earnings from ages 23 to 64 for associate degrees and 22 to 64 for certificates. We discount all estimates at a three percent rate to the year in which the student is 20. We also assume that earnings are zero while the student is enrolled in school (at age 21 for certificates and at ages 21 and 22 for associate degrees). The sum of earnings for all of these ages is equal to the typical lifetime earnings that people with each credential can expect.
Counterfactual earnings
Estimated earnings are only part of the ROI equation. It is also necessary to consider what students would have earned if they had not pursued an associate degree or certificate. The difference between these two values — the estimated earnings and counterfactual earnings — is the financial benefit of the credential. It is naïve, however, to simply use the typical earnings of a high school graduate as the counterfactual; people who choose to pursue postsecondary education differ in many ways from those who make different choices.
Therefore, we calculate counterfactual earnings to take account of the various observable differences between high school graduates and those who earned a sub-baccalaureate credential. The counterfactual takes stock of factors such as gender, race, ethnicity, location, urbanicity, ability, motivation, health, and family background. It is likely that we have not fully accounted for all unobservable differences, but our estimates of counterfactual earnings are certain to be closer to the true figure than the unadjusted average earnings of high school graduates. We calculate different counterfactual earnings for each Scorecard observation to take account of demographic and other differences between students enrolled in schools and programs.
To calculate counterfactual earnings, we first return to the 2009–2019 ACS sample. We restrict the sample to individuals with at least a high school degree but less than one year of college, with no higher degree. We drop people younger than 22 or older than 65 (effectively producing a sample of earnings for people aged 21 to 64). To align with the ACS sample of college graduates, we also drop people who are unemployed or not in the labor force, people enrolled in school or college, and people whose prior year income was less than $10,000.
We use the ACS sample to build an OLS regression model that predicts an individual’s log personal income based on age, sex, race, ethnicity, state, and metropolitan area. We use this model to predict counterfactual earnings for each Scorecard observation based on the racial and gender breakdown of the program and the location of the institution.
The Integrated Postsecondary Education Data System (IPEDS) at the National Center for Education Statistics collects data on the racial and gender breakdown of associate degree and certificate earners at America’s colleges and universities. Our model adjusts counterfactual earnings based on the race and gender distribution of program graduates as reported in IPEDS. The model also adjusts counterfactual earnings based on the school’s location (state and metropolitan area), on the assumption that counterfactual earnings are a function of the labor market opportunities available to high school graduates in the school’s geographic area. Community colleges in New York City will have higher counterfactual earnings than community colleges in rural Mississippi, since wages in New York City are higher.
Among the 17,500 Scorecard observations with reportable earnings data, 763 do not match to IPEDS. We are forced to drop these observations. Fortunately, these account for just four percent of the enrollment-weighted observations in the Scorecard. There are also over 1,000 certificate programs and just over 100 associate degree programs that lack demographic information on completers; we fill in the race and gender breakdown for these programs with the averages for the applicable credential level.
Returning to NVCC’s design and applied arts program as an example, for this observation the model predicts counterfactual earnings for a high school graduate living in the state of Virginia and the Washington, D.C. metropolitan area. The model weights the earnings of female high school graduates more heavily (the program is 58 percent female) and also adjusts the counterfactual based on the racial and ethnic breakdown of program graduates.
The regression model provides an estimate of counterfactual earnings after adjusting for basic demographics and geography. But there are many other factors that influence counterfactual earnings, including academic ability and family background. ACS, however, does not collect data on most of those factors.
Instead, we turn to the National Longitudinal Survey of Youth, 1997 cohort (NLSY97). NLSY97 is a nationally representative sample of 8,984 individuals born between 1980 and 1984. These individuals were first surveyed in 1997, when most of them were in high school, and were periodically re-contacted afterwards. The last follow-up with available data was in 2017, when NLSY97 respondents were between 33 and 37. In addition to basic data on demographics, education, and income, NLSY97 collects comprehensive information on many other aspects of each individual’s background and identity.
To exploit the NLSY97’s rich suite of variables, we follow a strategy used by Doug Webber (2014). First, we regress the log of respondents’ income on basic demographic information and a set of binary variables for several categories of educational attainment. The coefficients on these binary variables represent the “unadjusted” earnings premium associated with each education category.
Unfortunately, the small sample size of NLSY97 means we can only examine educational attainment within broad categories. These categories are: associate degrees in nursing and health; associate degrees in science and engineering; associate degrees in business; and all other associate degrees. NLSY97 does not separately identify individuals with undergraduate certificates, so we are unable to replicate this analysis for undergraduate certificate programs.
The regression excludes people who have less than a high school education and those with a bachelor’s degree or higher. To align our sample with ACS and the Scorecard, we exclude individuals earning less than $10,000 and those enrolled in school.
We then run a second regression of income on the demographic and educational attainment variables, but we also include a rich set of controls for ability, self-perception, and family background. The coefficients on the educational attainment variables represent the “adjusted” earnings premium associated with each education category; in other words, the portion of the educational earnings premium that cannot be explained by measured ability, family background, or other observable factors. Consequently, the difference between the educational attainment coefficients on the first and second regressions represents the portion of the education premium that is attributable to these factors.
The additional controls in the second regression include:
- Family background characteristics. Household income while the student was in high school, parents’ highest education level, and indicators for the absence of one or both parents.
- High school characteristics as reported by the student. The share of students who plan on attending college, the share of students who cut class, the quality of the teachers, and the frequency of disruptive incidents in school.
- Personal characteristics as reported by the student or her parents. Health conditions, substance use, and a general indicator for experiencing “hard times” as a child.
- Personality traits. The degree to which the student considers herself organized, conscientious, dependable, thorough, agreeable, cooperative, flexible, and trustful.
- Measures of ability. The student’s scores on the Armed Services Vocational Aptitude Battery — a standardized test with more comprehensive subject matter than the SAT or ACT, often used as a proxy for ability in the academic literature.
We take the difference between the coefficients on the first and second regressions for each education category and add these “adjustment factors” to our estimates of counterfactual earnings. Since the NLSY97 surveys its respondents several times, we can run the regressions for different age categories to obtain different adjustment factors for ages 21 to 34. Since NLSY97 respondents were last surveyed during their mid-thirties, we must assume that the adjustment factor remains constant for the rest of the career.
The table below displays the results of the regressions for each age group and educational attainment category. The reference category is high school graduates.
For undergraduate certificates, we assume an adjustment factor of zero. This is a strong assumption, to be sure, but one we believe to be reasonable given that the adjustment factors for associate degrees are already quite low (between 0.03 and 0.07 log points) and the fact that the vast majority of certificate-granting institutions are open-enrollment, which should minimize selection effects on earnings. Still, the reader should bear in mind that this assumption may result in an underestimate of counterfactual earnings (and hence an overestimate of ROI) for certificate programs.
Adding the adjustment factors to the estimates of counterfactual earnings derived from the ACS regression analysis yields our final estimates of counterfactual earnings. Before making the adjustment, counterfactual earnings for NVCC’s design program during the first year of enrollment (at age 21) are 10.17 ($26,052). But after we add the adjustment factor of 0.027 for the appropriate age and degree category, counterfactual earnings rise to 10.20 ($26,772). The model produces separate counterfactual earnings estimates for each stage of students’ careers; for instance, counterfactual earnings for NVCC’s design program at age 45 are $45,514.
We subtract counterfactual earnings from estimated earnings to generate an estimate of the boost in lifetime earnings that results from graduating with a particular degree from a particular college. To compute the earnings boost, we discount all estimated and counterfactual earnings at a three percent rate to the year in which the student turns 20. For NVCC’s design program, discounted lifetime earnings are $1,082,878, while discounted counterfactual lifetime earnings are $961,007 (which includes the two years while the student is enrolled in school). The lifetime earnings boost associated with the degree is the difference between earnings and counterfactual earnings, or $121,872.
The cost of associate degrees and certificates
The final element of the ROI calculation is the direct cost of an associate degree or certificate. How much do the student and her family pay to receive the estimated boost in earnings associated with the credential? We estimate tuition after all sources of grant aid — federal, state, local, and institutional — but before student loan aid. We include the cost of textbooks and equipment in college costs, but not living expenses, as students must pay for the basic costs of living regardless of whether they attend college.
Data on tuition and financial aid is available in IPEDS, though IPEDS’ “net price” variable incorporates living expenses and is thus not suitable for our purposes. Instead, we construct our own estimate of net price based on published tuition rates and average financial aid. We take the sum of published tuition, required fees, and the estimated cost of books and equipment. We use in-state tuition for public community colleges that charge differential tuition by residency.
We subtract average financial aid from this measure of “sticker” costs. IPEDS reports the average amount of federal, state, local, and institutional aid provided to full-time students in their first year of enrollment who receive Title IV federal financial aid. (The variable incorporates only students paying the resident tuition rate for public institutions.) For comparability with the cohort of students in the College Scorecard, we use the average of tuition and aid figures from the 2015–16 and 2016–17 academic years.
In four-year programs, colleges tend to slash financial aid grants after the freshman year; net tuition therefore rises in the sophomore year and beyond. But for associate degree programs, data from the National Postsecondary Student Aid Study (NPSAS) indicates that there is, on average, no meaningful reduction in financial aid awards during the second year of associate degree programs. We therefore assume that net tuition is the same for both years (and subsequent years, if the student takes longer than two years to graduate).
A word of caution is in order about net tuition figures. Both Scorecard earnings data and IPEDS financial aid data reflect only students who used Title IV federal financial aid programs. Just 62 percent of students at public community colleges use federal aid (versus more than 70 percent at four-year institutions). Students who use federal aid may receive lower net tuition packages than non-aided students, leading to an understatement of net prices. This is less of a concern at private for-profit institutions, where the percentage of students receiving federal aid is close to 90 percent.
Moreover, IPEDS lacks data on sticker-price and average financial aid awards for some schools, mostly for-profit colleges that grant only undergraduate certificates. However, collection of data on net tuition revenue is more comprehensive. For Scorecard observations that lack IPEDS data on sticker prices and financial aid, we instead use net tuition revenue per full-time equivalent student. (The correlation between traditionally measured net tuition and net tuition revenue at institutions that report both is 0.77). Net tuition revenue per student is truncated at the 99th percentile. Twenty-five observations in the Scorecard remain without tuition information even after bringing in this alternate source of data; we drop these.
In 2015–16 and 2016–17, Northern Virginia Community College listed average real tuition costs of $5,622 and average real books and equipment costs of $1,937. The sum of these values ($7,559) is the price before aid. NVCC also provides an aid package equal to $4,733 on average, which we subtract from the price before aid. This yields NVCC’s net price of $2,826 per year. An associate degree from this college requires paying tuition for two years, making the full tuition cost of this degree $5,652 (before discounting).
We assume that undergraduate certificate students pay one year’s worth of tuition, while associate degree students pay two years’ worth. We discount all tuition payments at a three percent rate to the year in which the student turns 20 and subtract them from the estimated lifetime earnings boost associated with each credential. For NVCC’s design and applied arts program, this final calculation yields an ROI of $116,465.
Adjustment for completion outcomes
The benefits of an investment in higher education are almost entirely concentrated in the degree or certificate that students receive at the end of their studies. Realizing a positive return on investment is usually contingent on completion. The “clean” ROI calculation described above assumes that the student’s likelihood of on-time graduation is 100 percent. But fewer than half of college students complete their education in the “normal” time frame, and some never finish at all. Rates of non-completion are higher than average at public community colleges, which grant most of the country’s associate degrees.
We therefore compute an alternative measure of ROI that incorporates the differential likelihood of completion at different institutions. IPEDS records data on completion outcomes for first-time, full-time degree- or certificate-seeking students at the institution level. For bachelor’s degree-granting institutions, completion outcomes for sub-baccalaureate credentials are recorded separately.
Student counts are recorded for six different completion outcomes: complete within 100 percent of normal time, complete within 150 percent of normal time, complete within 200 percent of normal time, still enrolled after 200 percent of normal time, transferred out, and left without transferring. We calculate the percentage of students who end up in each of four categories: complete within 100 percent of normal time, complete within 150 percent of normal time, complete within 200 percent of normal time, and drop out. We count students who are still enrolled after 200 percent of normal time as dropouts. We exclude transfer students from both the numerator and the denominator of these completion outcome calculations, as most transfer students out of community colleges are seeking bachelor’s degrees, and the ROI calculations in this report are concerned with sub-baccalaureate credentials. For sub-baccalaureate programs at bachelor’s degree-granting institutions, we also exclude students who start out in a sub-baccalaureate program but end up earning a bachelor’s degree from both the numerator and the denominator.
Unfortunately, IPEDS does not distinguish between students who started out seeking an associate degree versus a certificate; there is just one institution-level set of completion outcomes. We must assume that completion rates are similar across all programs at the same institution.
Since completion rates for certificates are higher than those of associate degrees, calculating completion rates at the institution level will lead to overestimates of completion rates for associate degrees and underestimates of completion rates for certificates at institutions that grant large numbers of both types of credentials. Fortunately, most institutions specialize in either associate degrees or certificates, so this is not a concern for most Scorecard programs.
About 350 Scorecard programs do not have completion rate data recorded in IPEDS. We fill in completion outcomes data for these programs with the average for the same credential level and sector (public, private nonprofit, and private for-profit).
With completion outcome rates in hand, we compute ROI for each of the four outcomes. Students who complete within 100 percent of normal time are assigned the “clean” ROI for their program. Students who complete within 150 percent of normal time pay 50 percent more tuition and spend additional time out of the labor force (half a year for certificate students and a full year for associate degree students). Completing within 200 percent of normal time means paying 100 percent more tuition and spending even more time out of the labor force (an additional year for certificate students and two additional years for associate degree students). Taking extra time to graduate therefore reduces ROI on multiple fronts: students must pay more tuition, lose additional labor-market earnings, and enjoy the earnings benefits of their degrees for less time.
We assume that students who do not finish their programs spend 75 percent of the normal completion time pursuing the degree. Associate degree seekers spend a year and a half in school, paying the applicable tuition rates and losing out on labor market earnings during that time period. Certificate-seeking students spend nine months in school. We assume that dropouts receive none of the labor market benefits of the credentials they sought; in other words, their lifetime earnings boost is zero.
After computing ROI for each of the four completion outcomes, we compute a weighted-average ROI for each program using the institution’s actual completion data. For instance, based on IPEDS data we calculate that at NVCC, 13 percent of students complete a degree or certificate in 100 percent of normal time, 17 percent complete within 150 percent of normal time, eight percent complete within 200 percent of normal time, and 63 percent do not complete. (Recall that transfer students are not included in the denominator of these proportions.)
For NVCC’s associate degree in design, “clean” ROI for an on-time completer is $116,465. For a student who completes in 150 percent of normal time, ROI drops to $85,298; completing in 200 percent of normal time reduces ROI still further, to $51,738. Dropping out results in ROI of negative $43,282. The completion-adjusted ROI for NVCC’s design program ends up at $5,938.
Adjustment for full cost of education
Most students do not bear the full cost of their education. Government financial aid programs, such as Pell Grants, shift some of the burden to taxpayers. Public community colleges are heavily subsidized by state and local appropriations, and after incorporating financial aid, the average net consumer price at many schools is zero. While prospective students should be most interested in whether the boost in earnings from their degree justifies the tuition they paid, other stakeholders may be interested in whether this earnings increase justifies underlying spending.
We calculate a measure of ROI which accounts for underlying spending, not just tuition. This calculation essentially replaces net tuition with the college’s education-related spending per full-time equivalent student. Education-related spending is the sum of spending on instruction, academic support, student services, and institutional support (administration). It excludes spending on research and public services, as well as spending on auxiliary enterprises such as dormitories and independent operations such as hospitals.
At NVCC, education-related spending per full-time equivalent student is $9,513, more than three times its net tuition of $2,826. Calculating ROI with respect to spending rather than tuition reduces “clean” ROI from $116,465 to $103,669; ROI after adjusting for completion outcomes is reduced from $5,938 to negative $6,868.
Caveats
We have endeavored in this report to get as close to a measure of the true return to sub-baccalaureate credentials as possible. Yet the exercise still requires making several assumptions that may not hold in reality. In the interests of full transparency, we have listed the most important caveats below, organized by their implications for our estimates of ROI.
Caveats that should generally lead to overestimates of ROI:
- The variables in NLSY97 probably do not fully control for all sources of selection bias between associate degree completers and high school graduates. Moreover, we are not able to make selection bias adjustments for certificate programs. These limitations lead us to underestimate counterfactual earnings.
- The College Scorecard reports earnings for Title IV-recipient students only, and our estimates of net tuition incorporate average aid for Title IV students, not all students. Since Title IV students receive more aid, this leads to an understatement of net tuition for the “average” student.
- We assume that each student attends school close to the beginning of her adult life and works until retirement. But many students pursue higher education later in life and have fewer years to put their credential to use in the labor market. This is especially true for students who pursue undergraduate certificates. ROI for older students will be significantly lower than the estimates of ROI we provide here.
Caveats that should generally lead to underestimates of ROI:
- Scorecard earnings data only capture individuals who are working. For consistency, we exclude unemployed people from our ACS-derived estimates of earnings and counterfactual earnings. Unemployment rates are lower among people with some college experience than they are among high school graduates, though it is unclear how much of this is due to selection effects. Our estimates of ROI do not incorporate likelihood of unemployment — only earnings for people who do have a job. This limitation will lead to an underestimate of ROI.
- Many students use an associate degree as an intermediary credential on the road to a bachelor’s degree. If a student applies her credits from the associate degree towards a bachelor’s degree, her ROI may be higher, since bachelor’s degree holders generally out-earn associate degree holders — though there are exceptions! However, the goal of this report is to estimate ROI for the associate degree (or certificate) alone; we do not account for the associate degree’s potential value as a stepping-stone credential. Those interested in the ROI of bachelor’s degrees should read our earlier work on the subject.
Caveats that could lead to an overestimate or underestimate of ROI:
- We assume that the quantile ranking for each program in the distribution of earnings for people with that credential remains constant throughout individuals’ careers. In reality, many people will move around on the earnings distribution during their lives, and there may be some degree of mean-reversion for programs at the extremes. Given that ACS does not classify holders of sub-baccalaureate credentials by field of study, the earnings estimates using this method are likely to be more imprecise that they are for bachelor’s degrees.
- We assume that all undergraduate certificate programs last for one year. But in reality, some last for six months or less, while others can last 18 months or more. We lack the data to accurately assign program durations to all certificates, so we assign all certificates a length of one year. This leads to an underestimate of ROI for short-term certificates and an overestimate for long-term certificates.
- Some data points are only available at the institution level. For instance, we must assume that completion outcomes are similar across all programs at the same institution. But if the completion rate for a certain program is higher than the average for its institution, our calculations will underestimate ROI for that program (and vice versa). This is especially a concern for institutions that offer both certificates and associate degrees; the former have higher completion rates, and so we will tend to underestimate ROI for certificates (and overestimate ROI for associate degrees) at institutions that offer both.
- At community colleges, it is common for students to attend part-time while working; however, this report assumes that students do not work and instead attend school full-time. Attending part-time while working means a smaller loss of labor market income while enrolled. However, it also means that students must wait longer to enjoy the benefits of their degrees, reducing the lifetime earnings gain. Accounting for these factors might raise or lower estimated ROI, on net.
- We do not account for the social benefits or social costs of sub-baccalaureate credentials. Well-educated workers can raise economy-wide productivity, meaning some individuals may not capture the full value of their education in higher earnings. But there are also social costs to postsecondary education, since a greater supply of workers with postsecondary credentials feeds credential inflation. This lowers salaries for non-college educated people. Accounting for these externalities may raise or lower “social” ROI.
Given the available data, there is no perfect way to evaluate the ROI of an associate degree or certificate. But the imperfect datasets and tools at our disposal allow a reasonable estimation of ROI, with caveats. As data collection on higher education outcomes improves, we should be able to assemble some of the missing puzzle pieces and provide even more accurate estimates of ROI.