How We Calculated the Return on Investment of a College Degree
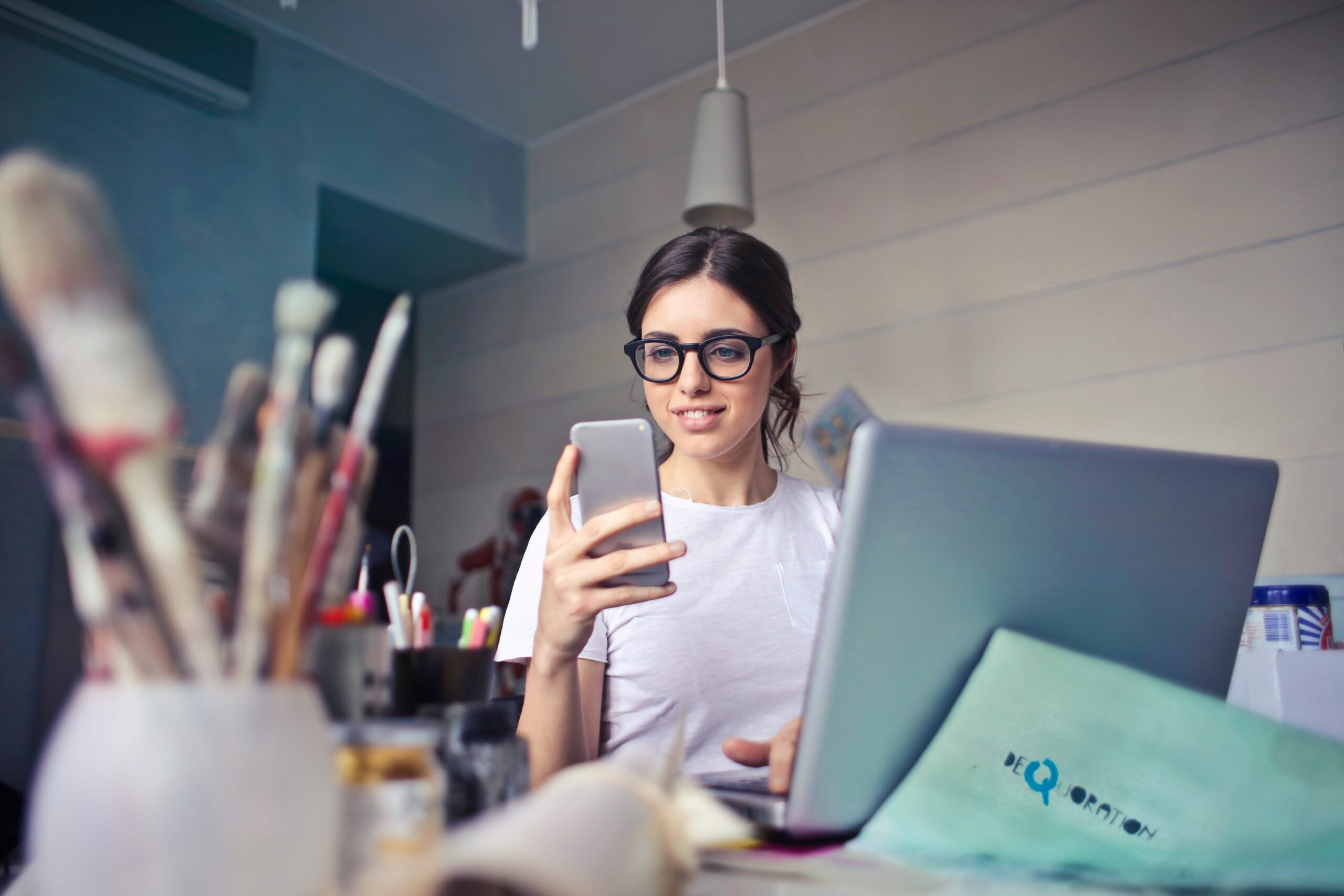
Photo by bruce mars on Unsplash
Our paper Is College Worth It? A Comprehensive Return-on-Investment Analysis calculates the net financial gains (increase in earnings minus education costs) that students can expect from thousands of bachelor’s degree programs across America. The project incorporates data from the U.S. Department of Education’s College Scorecard, the American Community Survey, the National Longitudinal Survey of Youth, and the Integrated Postsecondary Education Data System to build an interactive database aimed at helping students make better choices regarding whether to attend college, where to go, and what to study.
This page describes the methodology behind the construction of the ROI database. The ROI of a college degree combines three elements:
- Estimated Earnings: The amount that a student who graduates with a particular bachelor’s degree can expect to earn over the course of her life.
- Counterfactual Earnings: The amount that the same student would have earned over the course of her life had she not gone to college. This number includes the income that the student would have earned while she was in school.
- College Costs: The amount that the student spends on tuition, required fees, and other education-related expenses such as books and equipment. It does not include living expenses, as the student must spend money on lodging and food regardless of whether she goes to college.
ROI is equivalent to estimated earnings minus the sum of counterfactual earnings and college costs. I assume that the student attends college immediately after graduating high school at age 18. In the main specification, she attends college for four years, earns her bachelor’s degree, and enters the labor force at age 23. Her working career lasts 42 years, between ages 23 and 64. (Alternative specifications change some of these assumptions.) All cashflows are discounted at a real 3% rate to the year in which the student turns 18. Unless otherwise noted, I adjust all figures to 2020 dollars.
Estimated earnings
The U.S. Department of Education’s College Scorecard by Field of Study dataset provides information on the median earnings of college graduates for over 30,000 unique bachelor’s degree programs. Data are provided at the institution-program level: the dataset not only provides the difference in earnings between graduates of Pennsylvania State University and Swarthmore College, but also the difference in earnings between economics majors and political science majors who both attended Swarthmore.
Scorecard earnings are defined as the sum of wage and salary (Form W-2) income and positive self-employment income for individuals who received federal Title IV financial aid. Those who were not employed are excluded from earnings cohorts. Institution-program observations with small cohort sizes are suppressed for privacy reasons, so earnings data are not available for all 84,000 bachelor’s degrees listed in the Scorecard database. But the vast majority of students completed a program with reportable data: according to Scorecard documentation, just 14% of federally-aided bachelor’s degree recipients were in a program with privacy-suppressed data.
The Scorecard’s central limitation is that it only measures student earnings in the first two years after graduation. But since wage profiles tend to be steep during the early career, Scorecard earnings may significantly underestimate the lifetime returns to a college degree. For that reason, I augment the Scorecard figures with data from the American Community Survey (ACS), provided by the IPUMS USA database at the University of Minnesota.
Conducted by the U.S. Census Bureau, the ACS surveys a random 1% sample of the U.S. population every year, collecting basic demographic data along with information on individuals’ earnings. Since 2009, the ACS has also asked bachelor’s degree holders about their undergraduate college major. The ACS does not, however, ask respondents where they attended college.
To estimate life-cycle earnings for Scorecard programs, I use a subsample of the ACS datasets for the years 2009 through 2019. (I exclude the 2020 sample, as disruptions to data collection during the Covid-19 pandemic may yield unreliable estimates.) The combined subsample includes individuals with a bachelor’s degree or higher. I exclude anyone younger than 24 or older than 65. (Earnings are reported for the previous year, so this effectively gives me a sample of incomes for people between ages 23 and 64.) To align with the cohort definition in the Scorecard, I also exclude those currently enrolled in school or college, people who are unemployed or not in the labor force, and anyone with real earnings below $10,000 per year (as this may signal nonemployment in the previous year, when earnings were measured). This yields a sample of 4.5 million observations.
I divide the sample into fourteen age groups of three years each, starting with ages 24 to 26 (corresponding to earnings at ages 23 to 25). Within each of the age groups, I calculate the mean and standard deviation of the natural log of personal income for each of the 373 unique college majors in the Scorecard. This yields an estimate of average earnings over the career for each major. The steep wage profiles for many college graduates are apparent. For economics majors, mean earnings at ages 23 to 25 are $52,635; by ages 44 to 46, mean earnings rise to $97,253.
One challenge concerns individuals with graduate education, as I must disaggregate the portion of their earnings attributable to the graduate degree versus the bachelor’s degree. Simply excluding them from the analysis would bias my estimates, since individuals who choose to go to graduate school may have higher earnings potential than peers with the same major who earn only a bachelor’s degree.
I assume that 80% of the difference between the average earnings of people with bachelor’s degrees and people with graduate degrees (within each age/undergraduate major category) is attributable to the graduate degree, in line with the results of Altonji & Zhong (2021). In other words, I subtract 80% of the difference between average graduate degree earnings and average bachelor’s degree earnings for each age/major group from the earnings of all individuals with graduate education. I do this individually for each level of graduate degree (master’s, professional, and doctoral).
After calculating mean earnings for each major, I compare individual Scorecard observations to ACS mean earnings for the relevant major category within the 23-to-25 age group, which roughly corresponds to the ages at which Scorecard earnings are assessed. Specifically, I calculate a Z-score for each Scorecard observation. The Z-score is equivalent to the log difference between the individual Scorecard earnings and the ACS mean earnings for that major, divided by the ACS standard deviation for that major. The Z-score is a measure of how abnormally high or low a Scorecard observation is relative to the average for that major.
Consider the following example, using my alma mater (Swarthmore College) and my undergraduate major (economics).
For the economics program at Swarthmore College, the College Scorecard reports that earnings one year after graduation are $70,390 and earnings two years after graduation are $82,707. Average Scorecard earnings for this observation are therefore $76,549 (11.246 in natural log terms). ACS mean earnings for economics majors at ages 23 to 25 are $52,635 (10.871 in log terms), and the ACS standard deviation for this group, in log terms, is 0.612. The Z-score for Swarthmore’s economics program is equal to (11.246–10.871)/0.612 = 0.612. In other words, earnings for Swarthmore economics majors are 0.612 standard deviations above the average for all economics majors.
I repeat this process for all programs with data in the Scorecard. The only exceptions are programs which have fewer than 50 corresponding observations in the 23 to 25 age group in ACS, as I judge that the sample size is too small to yield reliable estimates. I do not calculate ROI for these observations.
Weighted by enrollment, the average Scorecard program has a Z-score of 0.057. This suggests that Scorecard earnings slightly outpace the ACS averages for their corresponding majors. The likely explanation is the fact that the ACS sample includes all years between 2009 and 2019, while Scorecard earnings were measured in 2017 and 2018 only. The latter were economic boom years, so we would expect Scorecard earnings to be slightly higher than the ACS average. Still, the fact that the weighted-average Z-score is quite close to zero suggests that ACS is a reliable proxy for the earnings of the student population captured in the Scorecard.
Having calculated Z-scores for all Scorecard observations, I use the ACS mean and standard deviation for other age groups to generate earnings estimates for each Scorecard program at all points during students’ careers. I assume that median earnings for each program retain the same Z-score (relative to ACS mean earnings for that major) for all age groups throughout the life-cycle.
Continuing with the Swarthmore economics example, recall that the Z-score for this observation was 0.612. The key assumption is that typical earnings for Swarthmore economics majors will remain 0.612 standard deviations above the mean for all economics majors throughout students’ careers. For the 26-to-28 age group, ACS mean earnings for economics majors are $64,588 (11.076 in log terms) and the ACS standard deviation (in logs) is 0.645. Estimated earnings for Swarthmore economics majors at ages 26 to 28 are equal to 11.076 + (0.612*0.645) = 11.471 in log terms, and $95,877 in dollar terms. I repeat this process for every age group until retirement.
The calculations yield estimates of average earnings (within three-year age bands) from ages 23 to 64 for every institution-program observation in the College Scorecard with reportable earnings data. I discount all estimates at a 3% rate to the year in which the student is 18. I also assume that earnings are zero between ages 19 and 22, while the student is enrolled in school.
Counterfactual earnings
A reasonable estimate of college ROI would not simply compare the earnings of college graduates to the earnings of those with only a high school degree; such an estimate would be biased upward for many reasons. Those who choose to go to college — and especially those who complete college — differ in key ways from those who never earn a higher degree. In the academic literature, rigorous causal estimates of the financial return to college tend to lag the raw earnings gap between college graduates and high school graduates (though the causal return to college is still substantial). In other words, if college graduates had not gone to college, they would have still earned significantly more than the average high school graduate.
I therefore adjust counterfactual earnings to take account of the various demographic and geographic differences between high school graduates and college graduates. I also adjust for other factors that may correlate with both earnings and college attendance, such as cognitive ability, motivation, health, and family background. It is likely that I have not fully accounted for all unobservable differences, but my estimates of counterfactual earnings are certain to be closer to the true figure than the unadjusted average earnings of high school graduates. I calculate different counterfactual earnings for each Scorecard observation to take account of demographic and other differences between schools and programs.
To calculate counterfactual earnings, I return to the 2009–2019 ACS sample. I restrict the sample to individuals with at least a high school degree but no more than one year of college, with no higher degree. I drop people younger than 20 or older than 65 (effectively giving me a sample of earnings for people aged 19 to 64). To align with the ACS sample of college graduates, I also drop people who are unemployed or not in the labor force, people enrolled in school or college, and people whose prior year income was less than $10,000. These exclusions yield a sample of 5.5 million individuals.
I use the ACS sample to build an OLS regression model that predicts an individual’s log personal income based on age, sex, race, ethnicity, state, and metropolitan area. I use this model to predict counterfactual earnings for each Scorecard observation based on the racial and gender breakdown of the program and the location of the college.
The Integrated Postsecondary Education Data System (IPEDS) at the National Center for Education Statistics collects data on the racial and gender breakdown of bachelor’s degree recipients at America’s colleges and universities. The College Scorecard also provides the gender breakdown for 13,000 bachelor’s degree programs with sufficiently large cohort sizes to avoid privacy suppression. My model adjusts counterfactual earnings based on the gender breakdown of each program, or the gender breakdown of the school if program-level gender data are not available. The model also adjusts each observation’s counterfactual earnings based on the school’s racial and ethnic composition; this relies on the assumption that racial and ethnic diversity is similar across all programs at the same school.
The model also adjusts counterfactual earnings based on the school’s location (state and metropolitan area). High school graduates in New York City tend to earn more than high school graduates in rural Alabama; therefore counterfactual earnings for colleges in New York City are higher than colleges in rural Alabama. This adjustment assumes that colleges tend to draw students from the surrounding geographic area; survey data shows that most students attend college within 100 miles of home and around three-quarters stay within their home state.
Returning to the Swarthmore economics example, for this observation the model predicts counterfactual earnings for a high school graduate living in the state of Pennsylvania and the Philadelphia-Camden-Wilmington metropolitan area, where Swarthmore is located. This fictional individual’s earnings are weighted based on gender (the economics program is 37% female) and race/ethnicity (Swarthmore graduates are 50% white, 18% Asian, 16% Hispanic, 7% Black, and 9% other). The model yields separate estimates for each stage of the career, including while students are enrolled in college. For instance, counterfactual earnings at age 20 are $22,395; counterfactual earnings at age 45 are $44,728.
The regression model provides an estimate of counterfactual earnings after adjusting for basic demographics and geography. But there are many other factors that influence counterfactual earnings, including ability and family background. The ACS, however, does not collect data on most of those factors.
Instead, I turn to the National Longitudinal Survey of Youth, 1997 cohort (NLSY97). The NLSY97 is a nationally representative sample of 8,984 individuals born between 1980 and 1984. These individuals were first surveyed in 1997, when most were in high school, and periodically re-contacted afterwards. The last follow-up with available data was in 2017, when NLSY97 respondents were between 33 and 37. In addition to basic data on demographics, education, and income, NLSY97 collects comprehensive information on many other aspects of each individual’s background and identity.
To exploit the NLSY97’s rich suite of variables, I follow a strategy used by Doug Webber (2014). First, I regress the log of respondents’ income on basic demographic information and a set of binary variables for several categories of educational attainment. The coefficients on these binary variables represent the “unadjusted” earnings premium associated with each education category.
Unfortunately, the small sample size of NLSY97 means I can only examine educational attainment within broad categories. These categories are: bachelor’s degrees from public universities in engineering and computer science, other physical sciences, business and management, social sciences, health, education, humanities and other majors; bachelor’s degrees from private nonprofit universities in the physical sciences, business, social sciences and education, humanities and other majors; and bachelor’s degrees from private for-profit universities in any major. I also include a dummy variable for individuals who have an associate’s degree. To align my sample with the ACS and the College Scorecard, I exclude individuals earning less than $10,000, those enrolled in school, and those with less than a high school education. I also adjust the earnings of people with graduate education as I did for the ACS sample.
I then run a second regression of income on the demographic and educational attainment variables, but I also include a rich set of controls for ability, self-perception, and family background. The coefficients on the educational attainment variables represent the “adjusted” earnings premium associated with each education category — in other words, the portion of the return to education that cannot be explained by measured ability, family background, or other observable factors. Likewise, the difference between the educational attainment coefficients between the first and second regressions represents the portion of the education premium that is attributable to these factors.
The additional controls in the second regression include:
- Family background characteristics. Household income while the student was in high school, parents’ highest education level, and indicators for the absence of one or both parents.
- High school characteristics as reported by the student. The share of students who plan on attending college, the share of students who cut class, the quality of the teachers, and the frequency of disruptive incidents in school.
- Personal characteristics as reported by the student or her parents. Health conditions, substance use, and a general indicator for experiencing “hard times” as a child.
- Personality traits. The degree to which the student considers herself organized, conscientious, dependable, thorough, agreeable, cooperative, flexible, and trustful.
- Measures of ability. The student’s scores on the Armed Services Vocational Aptitude Battery (a standardized test with more comprehensive subject matter than the SAT or ACT, often used as a proxy for ability in the academic literature).
It is unlikely that these controls fully proxy for unobserved differences between high school and college graduates, but incorporating them should improve my estimates of counterfactual earnings. I take the difference between the coefficients on the first and second regressions for each education category and add these “adjustment factors” to my estimates of counterfactual earnings. Since the NLSY97 surveys its respondents several times, I can run the regressions for different age categories to obtain different adjustment factors for ages 23 to 34. Since NLSY97 respondents were last surveyed during their mid-thirties, I must assume that the adjustment factor remains constant for the rest of the career.
The table below displays the results of the regressions for each age group and educational attainment category. The reference category is high school graduates.
For example, the coefficient on the indicator for students with a bachelor’s degree in social sciences or education from a private nonprofit university is 0.139 on the first regression (without controls) and 0.046 on the second regression (with controls) for the 23-to-25 age category. The difference between these two values is 0.092, which means that the counterfactual earnings at ages 23 to 25 for all social science bachelor’s degrees at private nonprofit universities must be adjusted upwards by 0.092 log points to account for ability and family background.
Therefore, counterfactual earnings for Swarthmore economics majors at ages 23 to 25 rise from $29,246 (10.284 in log terms) to $32,074 (10.379 in log terms) after applying the adjustment factor. This yields my final estimates of counterfactual earnings (within three-year age bands) from ages 23 to 64 for all Scorecard observations with available data, as well as estimates of counterfactual earnings for ages 19 to 20 and ages 21 to 22.
I subtract counterfactual earnings from estimated earnings to generate an estimate of the boost in lifetime earnings that results from graduating with a particular degree from a particular college, as shown in the chart below for Swarthmore College economics majors. To compute the earnings boost, I discount all estimated and counterfactual earnings at a 3% rate to the year in which the student is 18. For Swarthmore economics majors, the earnings boost — or the estimated value of the college degree — is approximately $1.8 million over the course of the student’s career.
College costs
The final element of the ROI calculation is college costs: how much the student and her family must pay to receive the estimated earnings boost. I estimate tuition after all sources of grant aid — federal, state, local, and institutional — but before student loan aid. I include the cost of textbooks and equipment in college costs, but not living expenses, as students must pay for the basic costs of living regardless of whether they attend college.
Data on tuition and financial aid is available in IPEDS, though IPEDS’ “net price” variable incorporates living expenses and is thus not suitable for my purposes. Instead, I construct my own estimate of net price based on published tuition rates and average financial aid. I take the sum of published tuition, required fees, and the estimated cost of books and equipment. I use in-state tuition for public universities that charge differential tuition by residency.
I subtract average financial aid from this measure of “sticker” costs. IPEDS reports the average amount of federal, state, local, and institutional aid provided to full-time students in their first year of enrollment who receive Title IV federal financial aid. (The variable incorporates only students paying the in-state tuition rate for public universities.)
Because many universities slash financial aid grants after freshman year, I reduce first-year aid by a small amount to calculate aid for each subsequent year. According to the 2015–16 National Postsecondary Student Aid Study, in-state students at public universities receive 2% less aid in their sophomore year, 12% less aid in their junior year, 9% less aid in their senior year, and 42% less aid in any subsequent years. The aid reductions at private universities are comparable.
For comparability with the cohort of students in the College Scorecard, I use the average of tuition and aid figures from the 2015–16 and 2016–17 academic years. Tuition data is missing from IPEDS for 291 observations in the Scorecard. I discount all tuition payments at a 3% rate to the year in which the student turns 18.
Swarthmore College charged a sticker price of $53,340 during the relevant time period, inclusive of books and equipment costs. However, it also provided a financial aid package to Title IV students worth $47,895 per year, meaning net tuition costs for this group of students were just $5,445 on average. The four-year cost of tuition for Swarthmore economics majors is therefore $21,780 (before discounting).
A word of caution is in order about net tuition figures. Both Scorecard earnings data and IPEDS financial aid data reflect only students who used Title IV federal financial aid programs. Since students with greater financial need are more likely to apply for Title IV aid, and also receive larger aid packages from universities, net tuition for Title IV students is lower than net tuition for all students.
While a majority of college students use Title IV overall, the share at some highly selective colleges is much smaller. At Swarthmore College, for instance, just 11% of students use federal loans. Swarthmore’s tuition figures are therefore based on a minority of students who pay much lower net tuition than the typical student. In other words, the average Swarthmore student probably pays much more than $5,445 per year in tuition. Due to data limitations there is unfortunately no way to correct for this; it is simply a caveat the reader must bear in mind.
I subtract the present value of these tuition payments from the estimated lifetime earnings boost; for a Swarthmore economics degree, this yields an ROI of $1.7 million.
Adjustment for completion outcomes
An investment in higher education generally only pays off if the student actually receives a degree. But less than half of students graduate on time, and many don’t finish at all. For students uncertain about their chances of graduation, college is therefore a risky proposition. My main measure of ROI assumes that the student’s likelihood of on-time graduation is 100%, but this does not hold in reality. Therefore, I compute an alternative measure of ROI that incorporates the differential likelihood of completion at various institutions.
IPEDS reports data on completion outcomes for first-time, full-time students who begin college in a bachelor’s degree program, at the institution level. Student counts are reported for six different completion outcomes: graduate in four years with a bachelor’s degree, graduate in five years with a bachelor’s degree, graduate in six years with a bachelor’s degree, transfer out, remain enrolled after six years, and drop out entirely before six years have passed. (I exclude students in a seventh category, those who start in a bachelor’s degree program but leave college within six years with a lower credential such as an associate degree, who are generally very small in number.)
Since IPEDS only reports data at the institution level, I must assume that completion rates are similar across all programs at an institution.
I then compute ROI for four separate completion outcomes: finish a bachelor’s degree in four years, finish in five years, finish in six years, and drop out. Students who take five or six years to finish their degrees must pay five or six years’ tuition, stay out of the labor force for five or six years, and shorten their working careers by one or two years relative to someone who graduates on time.
According to the Beginning Postsecondary Students Longitudinal Study (BPS:12/17), the median bachelor’s degree student who doesn’t graduate within six years drops out after three years. Therefore, I assume the average dropout pays three years’ tuition, stays out of the labor market for three years, and receives no financial benefit from their college experience.
IPEDS data does not track what happens to students after they transfer out of an institution. Drawing on BPS:12/17 data once again, I assume that 31% of transfers graduate within four years, 21% graduate in five years, 16% graduate in six years, and 32% never finish their degrees. Likewise, I assume that one-third of students who are still enrolled after six years (generally a very small number) eventually finish their degrees.
I combine these probabilities and compute a weighted-average ROI across the four completion outcomes. In IPEDS, Swarthmore College reports that 88% of students graduate in four years, 4% in five years, and 2% in six years. Five percent of students drop out and a negligible number transfer or remain enrolled after six years. I calculate that lifetime ROI for a student who graduates from the Swarthmore economics program in four years is $1.7 million, but ROI drops to $1.6 million for a five-year graduation and $1.5 million for a six-year graduation. For dropouts, ROI is negative $126,000. The outcomes-adjusted ROI for the Swarthmore economics program ends up at $1.6 million.
Adjustment for full cost of education
Most students do not bear the full cost of their education. Government financial aid programs, such as Pell Grants, shift some of the cost to taxpayers. Public colleges and universities receive appropriations from state governments that enable them to charge subsidized tuition. Even at private colleges, gaps can exist between net tuition and the underlying cost of education, plugged by tax-advantaged donations and graduate programs. While prospective students should be most interested in whether the boost in earnings from their degree justifies the tuition they paid, other stakeholders may be interested in whether this earnings increase justifies underlying spending.
The measures of ROI which account for underlying spending essentially replace net tuition with the college’s education-related spending per full-time equivalent student in the ROI calculation. Education-related spending is the sum of spending on instruction, academic support, student services, and institutional support (administration). It excludes spending on research and public services, as well as spending on auxiliary enterprises such as dormitories and independent operations such as hospitals.
At Swarthmore College, net tuition is $5,445, but education-related spending per student is $72,534. Performing the ROI calculation based on spending rather than tuition reduces the economics program’s estimated ROI from $1.7 million to $1.5 million. If I also incorporate the adjustment for completion outcomes, ROI falls to $1.4 million.
Caveats
I have endeavored in this report to get as close to a measure of the true return to a college education as possible. Yet the exercise still required making several assumptions that may not hold in reality. In the interests of full transparency, I have listed the most important caveats below, organized by their implications for my estimates of ROI.
Caveats that should generally lead to overestimates of ROI:
- The variables in the NLSY97 probably do not fully control for all sources of selection bias between college graduates and high school graduates. This leads me to underestimate counterfactual earnings.
- Women who do not attend college are especially likely to work part-time voluntarily. Women who graduate college are much less likely to do so. Since counterfactual earnings for college-educated women are based on the earnings of non-college-educated women, the part-time phenomenon may lead to an underestimate of counterfactual earnings for college-educated women, who might be more likely to choose full-time work even if they had not attended college.
- I assume that living costs are the same regardless of whether the student decides to attend college. But students may pay more for living expenses if they attend college, particularly if they live in on-campus dormitories.
- The College Scorecard reports earnings for Title IV-recipient students only, and my estimates of net tuition incorporate average aid for Title IV students, not all students. Since Title IV students receive more aid, this leads to an understatement of net tuition for the “average” student.
- I assume that each student attends college at the very beginning of her adult life and works until retirement. But many students attend college later in life and have fewer years to put their degree to use in the labor market. ROI for these older students will be significantly lower in reality.
Caveats that should generally lead to underestimates of ROI:
- I assume that ACS-estimated earnings for today’s 35-year-olds are an accurate guide to what today’s 25-year-olds will earn ten years from now. But if earnings for college graduates increase faster than earnings for high school graduates, as some analysts predict, then my estimates of ROI will understate the return to college for today’s graduates.
- I attribute most of the premium that people with advanced degrees earn over peers with bachelor’s degrees to the graduate degree, not the bachelor’s degree. But preparation for a lucrative graduate degree may be part of the economic value of college for some students. This may lead to an underestimate of ROI, especially for fields with high graduate school attendance (such as biology and life sciences).
- Scorecard data only capture individuals who are working. For consistency, I exclude unemployed people from my ACS-derived estimates of earnings and counterfactual earnings. Unemployment rates are lower among college graduates, but my estimates of ROI do not incorporate likelihood of unemployment — only earnings for people who do have a job.
Caveats that could lead to an overestimate or underestimate of ROI:
- I assume that the Z-score for each program relative to average earnings for that field remains constant throughout graduates’ careers. In reality, many graduates will move around on the earnings distribution during their lives.
- I must assume that several data points available only at the institution level — namely graduation rates and racial/ethnic composition — are constant across majors. If the graduation rate for a particular program is higher than the graduation rate of its university, my figures will underestimate ROI for that program and vice versa.
- The limited sample size of the NLSY97 means I can only estimate the portion of the education premium attributable to ability and family background within broad educational categories. For instance, I can estimate these figures for all private nonprofit universities but not highly selective nonprofit universities. Since selective schools enroll high-ability students, this may lead me to underestimate counterfactual earnings for highly selective schools (and overestimate ROI). Similarly, I may overestimate counterfactual earnings for less selective schools and underestimate their ROI.
- I do not account for the social benefits or social costs of college. Well-educated workers can raise economy-wide productivity, meaning some individuals may not capture the full value of their education in higher earnings. But there are also social costs to college, since more college-educated workers feed credential inflation, which lowers salaries for non-college educated people. Accounting for these externalities may raise or lower estimated ROI.
Like the parable of the blind men and the elephant, calculating return on investment in higher education requires looking at data from various sources that reveal only pieces of the whole picture. My analysis knits together these various pieces of data to estimate ROI, but requires assumptions to do so. While a more comprehensive dataset would be ideal, researchers must work with the information we have. The caveats above should be regarded as the pieces of the puzzle we still have to fit together, which future data releases will hopefully allow us to assemble.